Subject archive for "data-science-leaders"
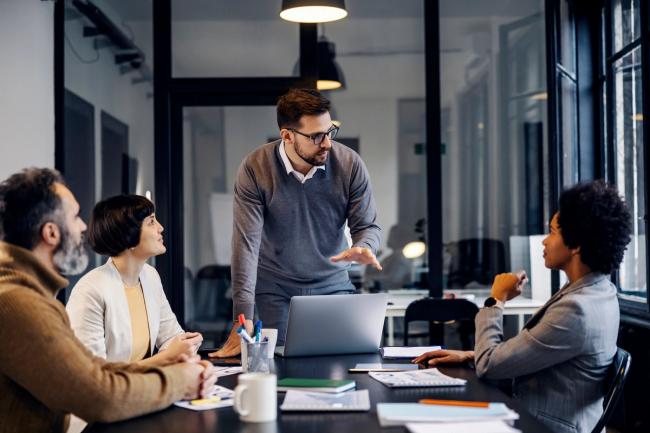
Mastering the art of AI leadership in times of transformation
Domino's Kjell Carlsson and guest speaker Forrester Research's visionary VP and Principal Analyst, Mike Gaultieri are here to help you answer these pressing issues. You can watch their insightful conversation on our upcoming webinar, "An expert's guide to surviving and thriving as an AI leader in a GenAI world."
By Domino3 min read
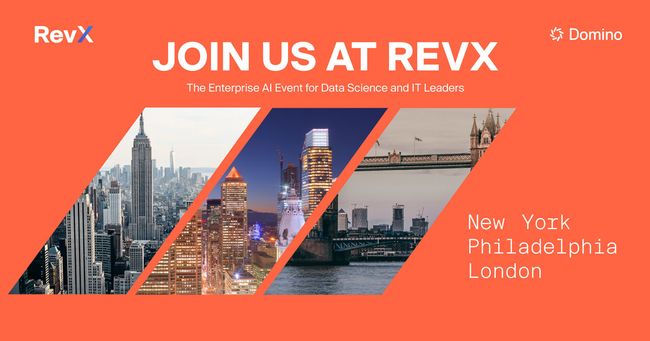
4 reasons why you need to be at RevX
The best hands-on learning experience for data science and IT leaders is a one-day free event called RevX. Reaching multiple cities, RevX will be headed your way, so you can discuss top-of-mind, vertical-specific topics related to AI and GenAI, and walk away with new, and thought-provoking strategies for implementation.
By Domino6 min read
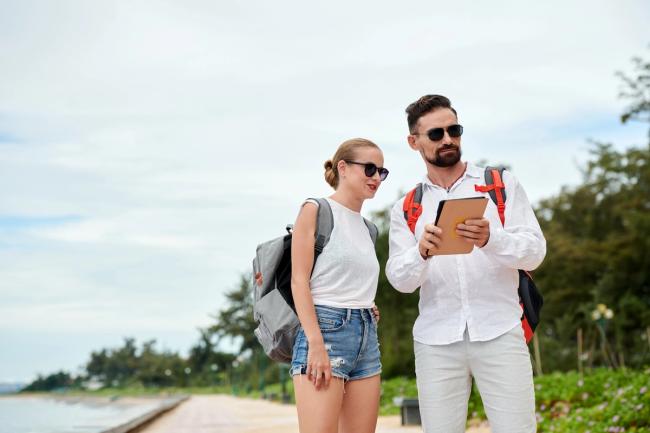
TripAdvisor's GenAI journey from billions of reviews to a virtual travel agent
See how TripAdvisor leverages GenAI to transform billions of reviews into a virtual travel agent tool.
By Yuval Zukerman5 min read
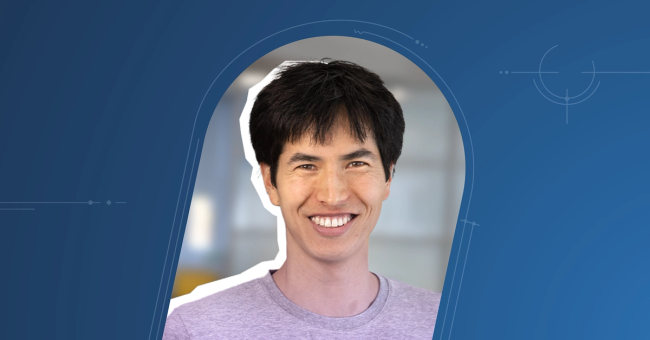
Ray Co-creator Robert Nishihara: How Easy Distributed Computing Changes Everything in Data Science
What if you wanted to do something really ambitious in data science–something like designing an innovative new search engine? Today, that would be a daunting task, and you’d probably need a big, highly qualified team of data scientists and programmers to bring your innovation to life. And you’d need months, if not years, to finish it.
By Lisa Stapleton3 min read
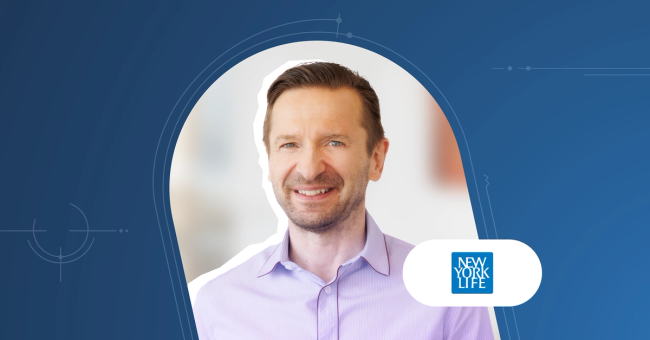
What the Rise of Data Science in Insurance Says About the Profession–and How It’s Changing
How do you build a data science capability into a powerful force for making decisions about virtually all facets of your business? And how do you recruit, train, organize, and retrain members of your team, in a field where competition for talent is intense and growing? These are just a couple of the questions that Glenn Hofmann, chief analytics officer at New York Life Insurance Company, has confronted and mastered in his tenure there.
By Lisa Stapleton4 min read
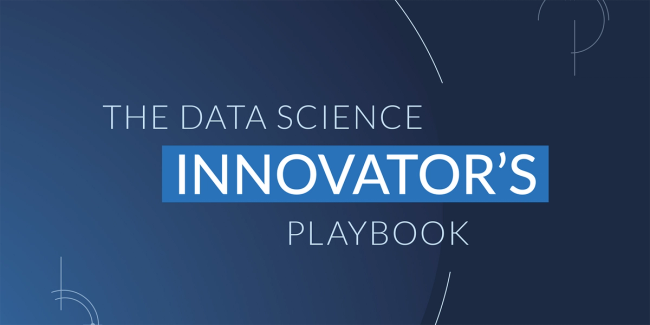
7 Top Innovators Share Insights, Trends and Career Advice in 'The Data Science Innovator’s Playbook'
Who’s doing the most innovative things in data science? Where is the profession going? And most importantly, what can you learn from some of the brightest in the business?
By Lisa Stapleton5 min read
Subscribe to the Domino Newsletter
Receive data science tips and tutorials from leading Data Science leaders, right to your inbox.
By submitting this form you agree to receive communications from Domino related to products and services in accordance with Domino's privacy policy and may opt-out at anytime.